As common as artificial intelligence has become in our daily lives — from Google’s Alexa, to Facebook’s data-driven ads, to Waze’s application of driver data to find the best route — it is little understood. The public tends to believe that AI comprehends complex problems and solves them the way humans do.
This myth can be blown up in an amusing fashion by reading posts at the blog aiweirdness, where Janelle Shane, an optics research scientist and AI researcher, writer, and public speaker, plays with machine learning algorithms to demonstrate that AI doesn’t get a lot of things humans instinctively grasp: candy heart messages, cat names (though “M. Tinklesby Linklater Soap” is oddly awesome), and most memorably, burlesque show names (“Deeptert!” and “Boodnass Tronpboons” stand out among the nonsensical weirdness).
Taking on three healthcare AI myths
In an article in Harvard Business Review, authors Derek A. Haas, Eric C. Makhni, Joseph H. Schwab, and John D. Halamka took on three myths of machine learning in healthcare.
- Myth #1: The perception that AI can replace doctors. While AI has an important role to play, for the foreseeable future AI will not replicate a doctor’s ability to provide care and treatment.
- Myth #2: Using “big data” in itself will lead to success. It’s true that more data is better, but only if it is the right data and it is fully understood.
- Myth #3: When an AI solution proves successful, it will be widely adopted and put to use. The fact is many powerful solutions are not accepted because they are not integrated into the workflow of potential users.
“The key is to be thoughtful about what types of problems AI is equipped to solve, who needs to be involved in developing the model and interpreting the output, and how to make it easy for people to utilize and act on the insights,” the authors say.
Make explicit decisions for the best outcomes
AllazoHealth’s AI engine is much more sophisticated than any of the open-source AIs that Janelle Shane uses. But it still needs precise directives, according to William Grambley, Chief Operating Officer of AllazoHealth, who told Bio Supply Trends that the key to getting the best results with AI is being very explicit about the outcomes being sought up-front.
So often in healthcare, the question is whether the outcome being sought is maximum health or cost-efficiency. “If you’re trying to solve for maximum health, then you’re going to have a different set of expectations from a program than if you’re trying to achieve the most cost effective outcome,” Grambley says. “If you don’t explicitly make a lot of decisions up front, it may not actually lead to what you’re trying to do.”
For example, Grambley points out that if a client was trying to avoid patient emergency room usage, there would be many ways of addressing that issue. “If you address it by somehow limiting access to it, that’s not the right answer. You have to address it in a way that supports overall health for people and better decision-making about where they go, and lots of other things.”
When AllazoHealth is working with clients, “we end up spending a lot of time asking those questions. I think, just broadly in healthcare [with regard to AI] if you aren’t explicit about what you’re trying to do, then you may run into issues,” notes Grambley.
About AllazoHealth
AllazoHealth uses artificial intelligence and a comprehensive data set of over 12 million lives to make a positive impact on individual patient outcomes. We optimize medication adherence and quality measures for pharmaceutical companies, payers, and pharmacies. Our AI engine targets individual patients with the right intervention, the right content, at the right time.
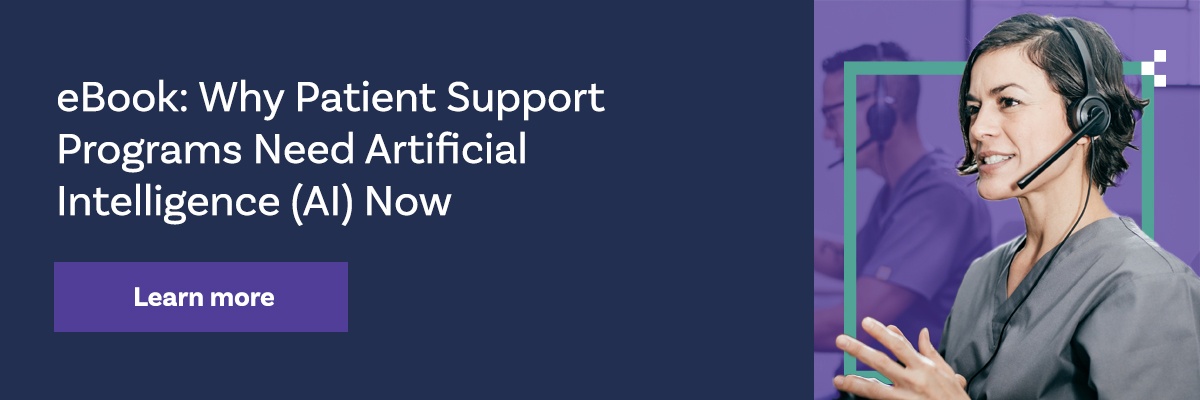